To establish an operational and integrated service at the iMagine platform for automatic processing of video imagery, collected by cameras at EMSO underwater sites, identifying and further analysing interesting images for purposes of ecosystem monitoring.
Aim
Development actions during iMagine
Using the iMagine platform for further developing of AI preselection of interesting images and of AI analysis of selected images for identification of biotaxa
Developing standards for managing and storage of the video imagery and annotated images from several EMSO sites in databases
Setting up an EMSO workflow at the iMagine platform with AI analysis service and connectivity of databases
Developing guidance documentation for RIs and RI nodes about standard data management practices and for using the AI analysis pipelines for biota classification
Reaching out to other EMSO sites, to LifeWatch, EMBRC and other relevant external users and providing support and training for adoption
Objective and challenge
The use case involves underwater video monitoring at three EMSO sites: EMSO-Obsea, EMSO-Azores, and EMSO-SmartBay. The aim is to establish an integrated service on the iMagine platform for the automatic processing of video imagery, enabling the identification and analysis of relevant images for ecosystem monitoring. By leveraging the capabilities of the iMagine platform and implementing AI models, the project aims to automate and enhance the analysis of underwater video imagery at the EMSO sites, facilitating scientific research and improving ecological understanding.
At the EMSO-Obsea site, there is significant unexploited image data collected from an underwater camera observing various fish species. Applying AI tools to these images would allow the extraction of valuable biological content, creating derived datasets that marine scientists can use to draw ecological conclusions. Manual analysis of the extensive dataset is time-consuming, and analyzing only a subset of the data would result in losing important information. Utilising the iMagine platform, a Deep Learning service will be trained and deployed to obtain species abundance data from existing and future images. These derived datasets will be crucial for studying species presence/absence over time and understanding changes in abundance in relation to environmental parameters, providing insights into the impact of climate change on the local fish community.
Timeline and progress
Objective and challenge
For the EMSO-Azores site, the imagery data collected by the observatory is being analyzed through the Deep Sea Spy platform, involving citizen participation. However, the data validation by experts is currently done manually and is time-consuming. Expanding the dataset with annotated and validated submarine images is essential for advancing marine science research. The iMagine AI platform will be used to develop and deploy AI models that can automatically annotate and validate images, improving the efficiency and accuracy of the process.
Timeline and progress
Objective and challenge
At the EMSO-SmartBay site, it is crucial to identify poor-quality video footage in real-time and within the Observatory Archive. Factors such as complete darkness, algal growth, suspended particulate matter reduction, and equipment failure can impact the utility of the observatory footage. Manual inspection of the video archive is time-consuming, and detecting interesting observations or occurrences, such as “Novelty” events or counting prawn burrows in the field of vision, is also laborious. The iMagine AI platform can aid in developing and deploying a service that enables quick detection of issues, efficient flagging and referencing of interesting footage, and the detection and enumeration of prawns and prawn burrows.
Timeline and progress
Image credit: Masayuki Agawa / Ocean Image Bank
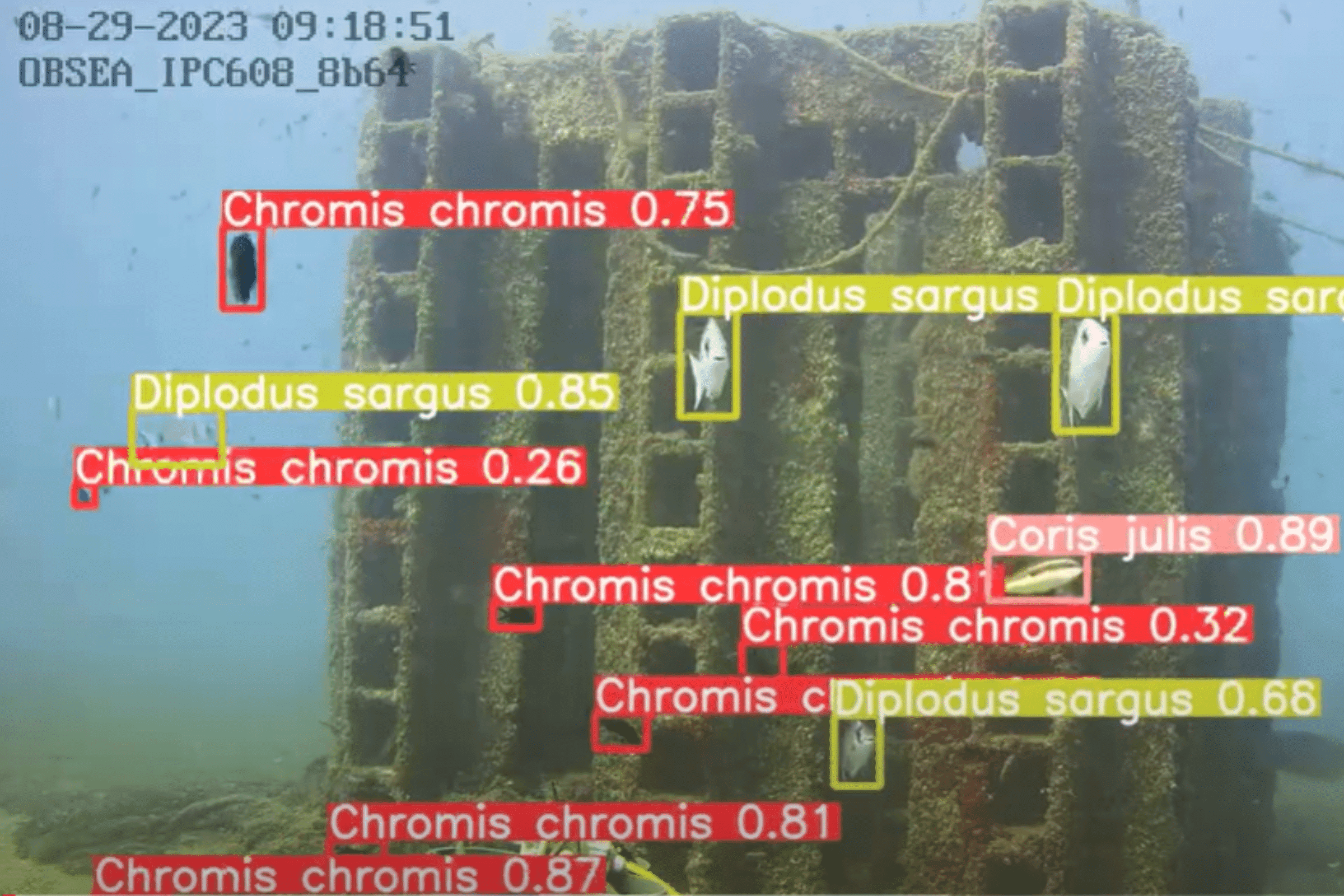