To develop, using the iMagine platform, a prototype service for processing acoustic underwater recordings for identification and recognition of marine species and other noise types (e.g., offshore piling).
Underwater Noise Identification
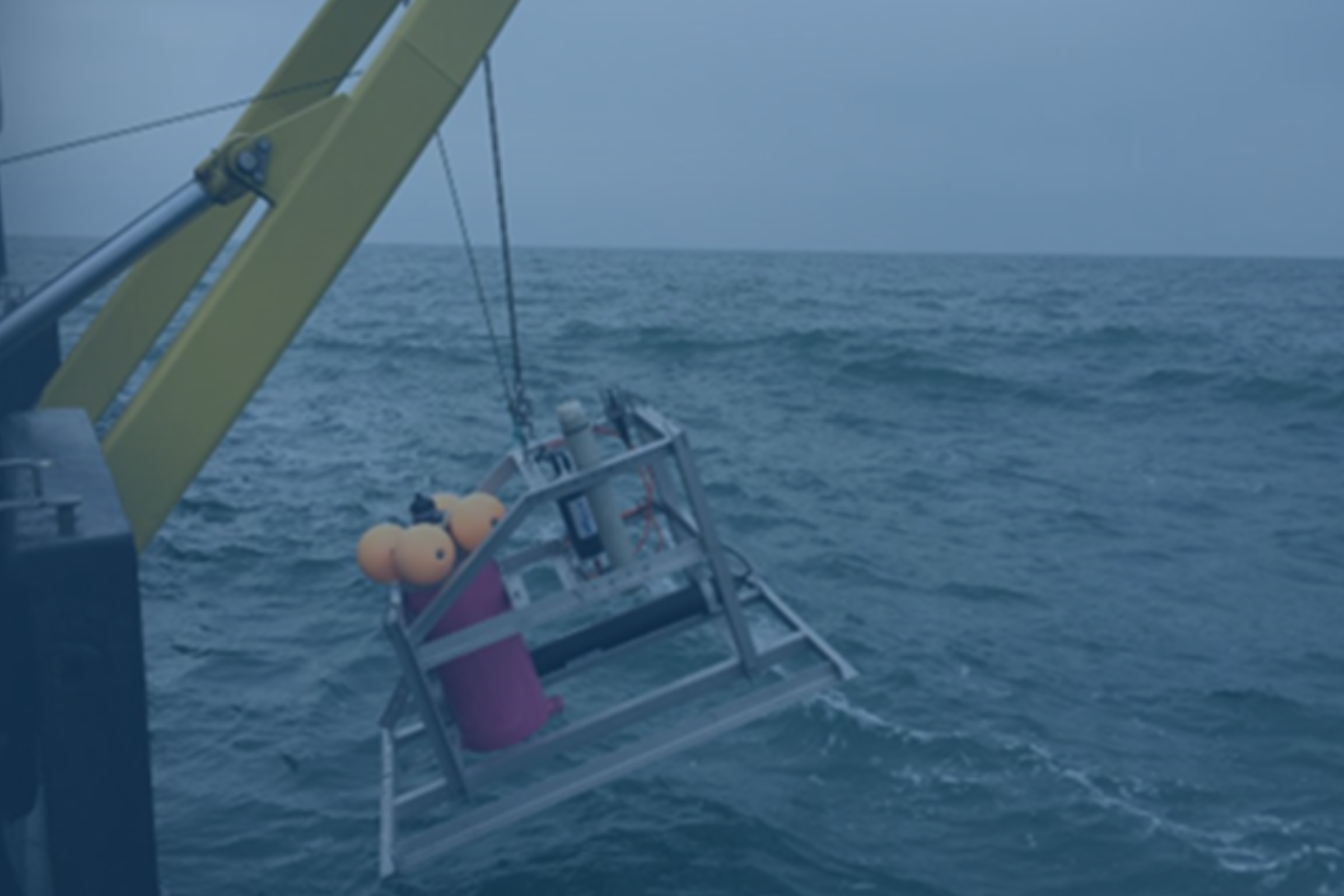
Aim
Development actions during iMagine
Objective and challenge
Underwater sound is crucial for aquatic life and survival. It consists of various sources, including living organisms, natural phenomena, and human-made noises. The European Union’s Marine Strategy Framework Directive (MSFD) recognizes underwater noise as a pollutant. In this context, the iMagine platform is being utilized to create a prototype service for analyzing acoustic underwater recordings. The goal is to identify and recognize marine species as well as other sound types, such as shipping noises.
Currently, there is a collection of 1.5 years’ worth of underwater sound data, with ongoing data collection efforts. However, the process of processing this data and identifying the sources is time-consuming, requiring significant individual effort. Furthermore, the existing process lacks automation. To address these challenges, the use case will leverage the iMagine AI platform and the project’s expertise to enhance data labeling and explore different AI techniques for sound recognition and identification.
Timeline and progress
Expected Results
The underwater sound classification service has large potential to support the scientific community in marine biodiversity and ecosystem research. Underwater sound and noise pollution are increasingly recognized as an essential indicator of healthy seas and oceans.
Detecting and classifying different species can contribute to species abundance assessment, and detection and characterization of noise pollution can provide insight in effects of human activities on marine life.