To develop, using the iMagine platform, a prototype diatom-based bioindication service using automatic pattern recognition algorithms from individual microscope images from freshwater environments.
Freshwater Diatoms Identification
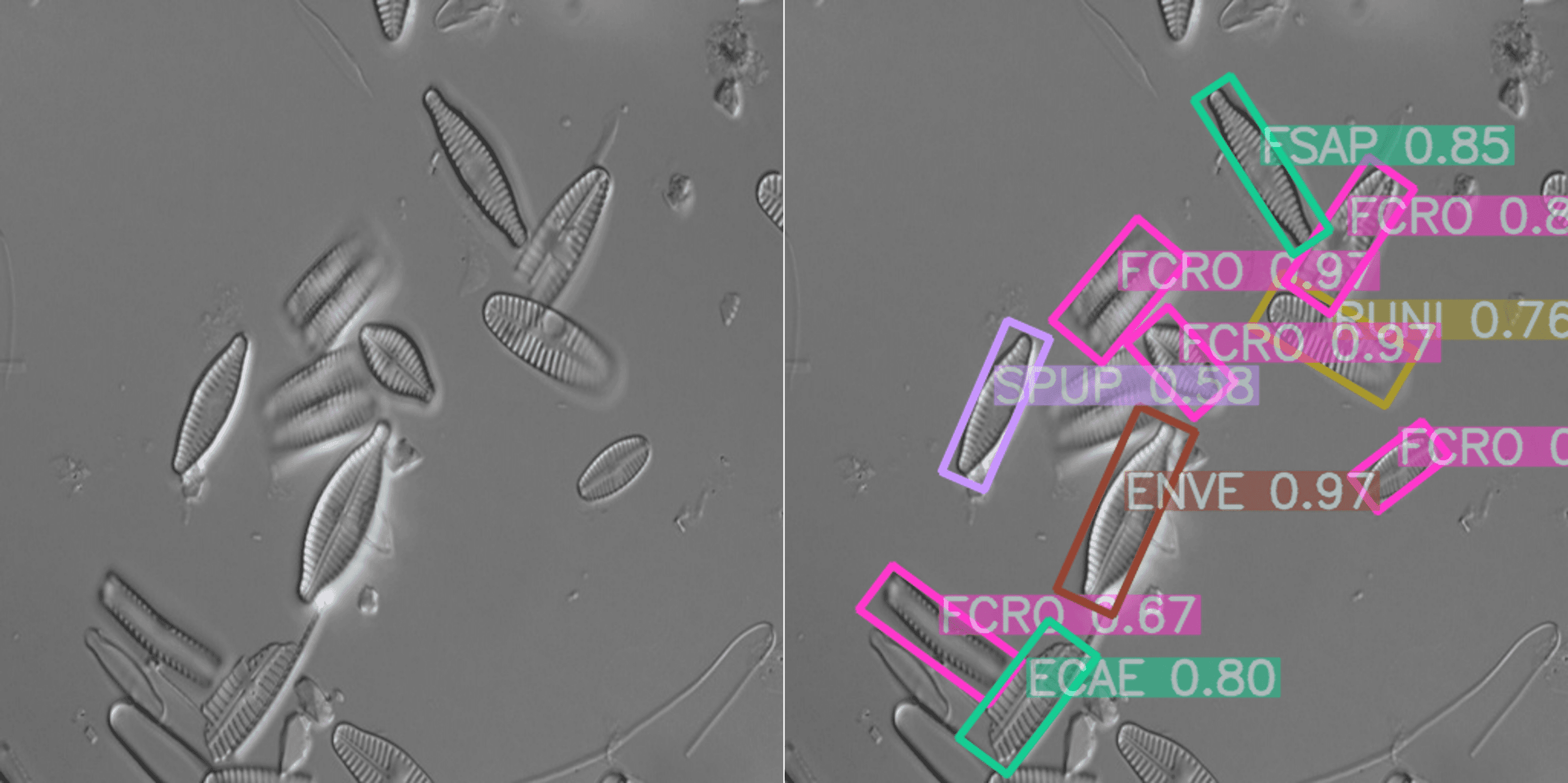
Aim
Development actions during iMagine
Setting up a development and training environment at the iMagine platform
Building a database for inserting and extracting of diatom images and gathering from various sources an extensive quality-controlled dataset of labelled (species name, traits estimation) light microscopy images for training and testing the CNNs
Analysis and training of AI models (CNN) fit for automatic classification of diatoms
Developing a GUI, using the iMagine platform, for user interaction
Documenting approach and experiences with resulting prototype
Contributing to dissemination and outreach, especially towards stakeholders (e.g., water agencies) and teachers (incl. for private consultancies)
Image database
Objective and challenge
Diatoms are unicellular microalgae found in various aquatic environments. They are commonly used as bioindicators to assess the ecological health of freshwater bodies like rivers and lakes, as mandated by the EU Water Framework Directive (WFD). The identification of diatom species relies on examining their silica exoskeletons under a microscope using classical light microscopy. However, quantifying important morphological features, such as size and deformations, which are crucial for bioindication, is currently a laborious and time-consuming task.
To address this challenge, the use case aims to develop a prototype diatom-based bioindication service that not only identifies diatom species but also quantifies key morphological features using automatic pattern recognition algorithms on microscope images. The iMagine AI platform will be leveraged for this development.
Currently, the identification of diatom species involves subjective and time-consuming manual processes, susceptible to biases related to operator experience and image quality. By standardizing and automating this process using AI, the accuracy and efficiency can be significantly improved.
Timeline and progress
Expected Results
Diatoms are unicellular microalgae present in all aquatic environments. They are routinely used as bioindicators for the ecological diagnosis of inland waters (rivers, lakes) as part of the implementation of the EU Water Framework Directive (WFD; Directive 2000/60/EC).
The development of high-throughput analyses will improve the diagnostic tools currently available, notably by improving the sampling effort, extending the current monitoring network to a larger number of stations and generating AI-based new metrics.
These improvements will have great potential all over Europe and foster the development of new AI-based bioindicators targeting other ecosystems (diatoms are present in all aquatic environments) and key bioindicators (e.g., benthic invertebrates).